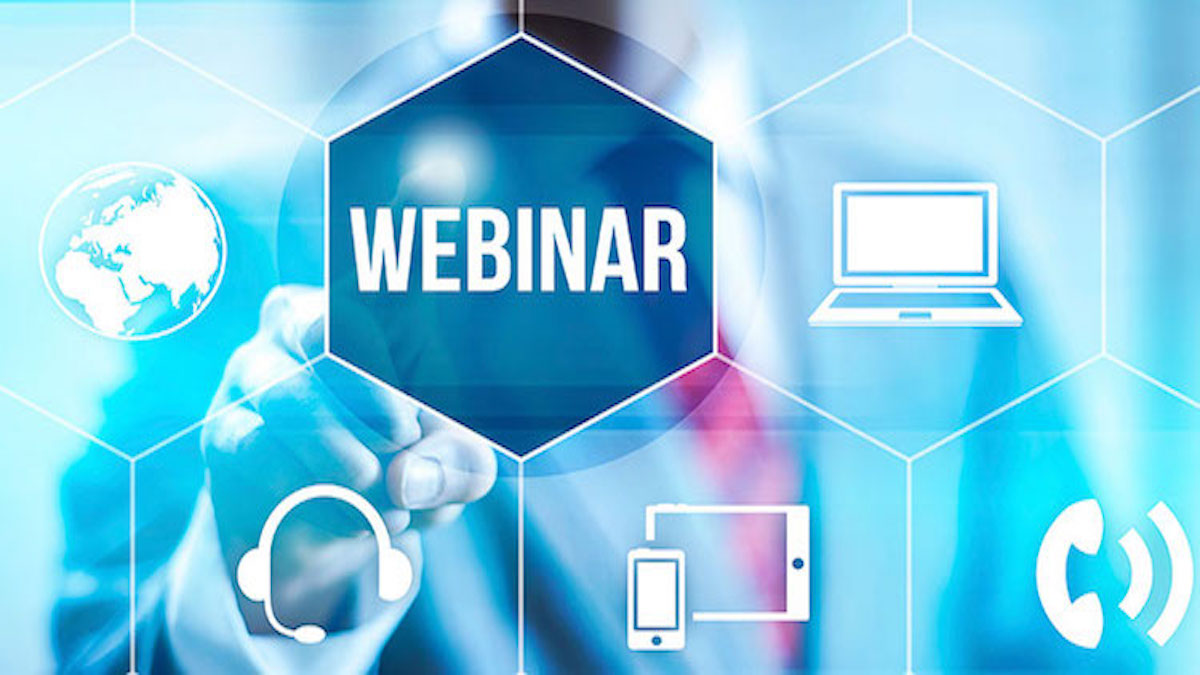
We are currently seeing exponential growth in the compute requirements for state of the art Deep Learning models as well as a rise in production environments for the application of these DL models as can be seen with the year starting out with Microsoft Turing Natural Language Generation model (17 billion parameters) and ending with Open AI GPT-3 (175 billion parameters).
Designing/choosing optimized AI infrastructure based on the use case (may it be hyperparameter search, large scale distributed training, production inference etc.) is becoming just as important as designing better DL models for the efficiency of an organization. The talk will include a discussion on the infrastructure choices that are available for deep learning and how the infrastructure setups are trying to keep up with the computing requirements.