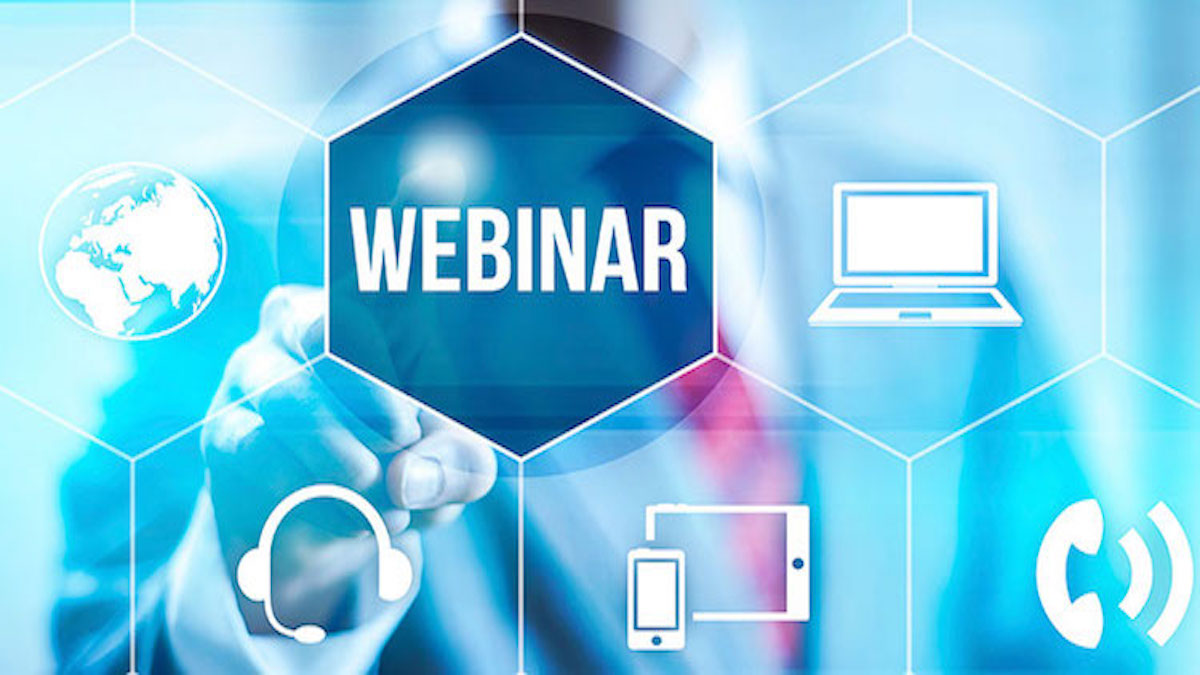
Machine learning (ML) is making incredible transformations in critical areas such as finance, healthcare, and defense, impacting nearly every aspect of our lives. Many businesses, eager to capitalize on advancements in ML, have not scrutinized the security of their ML systems.
Cyber-attacks can penetrate and fool AI systems. Trusted AI systems provide ability to detect and provide protection against adversarial attacks while understanding how issues with data quality impact system performance. With the rapid developments of artificial intelligence (AI) and deep learning (DL) techniques, it is critical to ensure the security and robustness of the deployed algorithms. Recently, the security vulnerability of DL (Deep Learning) algorithms to adversarial samples has been widely recognized. The fabricated samples can lead to various misbehaviors of the DL models while being perceived as benign by humans. Successful implementations of adversarial attacks in real physical-world scenarios further demonstrate their practicality. Hence, adversarial attack and defense techniques have attracted increasing attention from both machine learning and security communities and have become a hot topic in recent years. We will present the attack and defense method. We will also demonstrate these attacks on real life business models published on Public cloud and explain remediations one should consider.