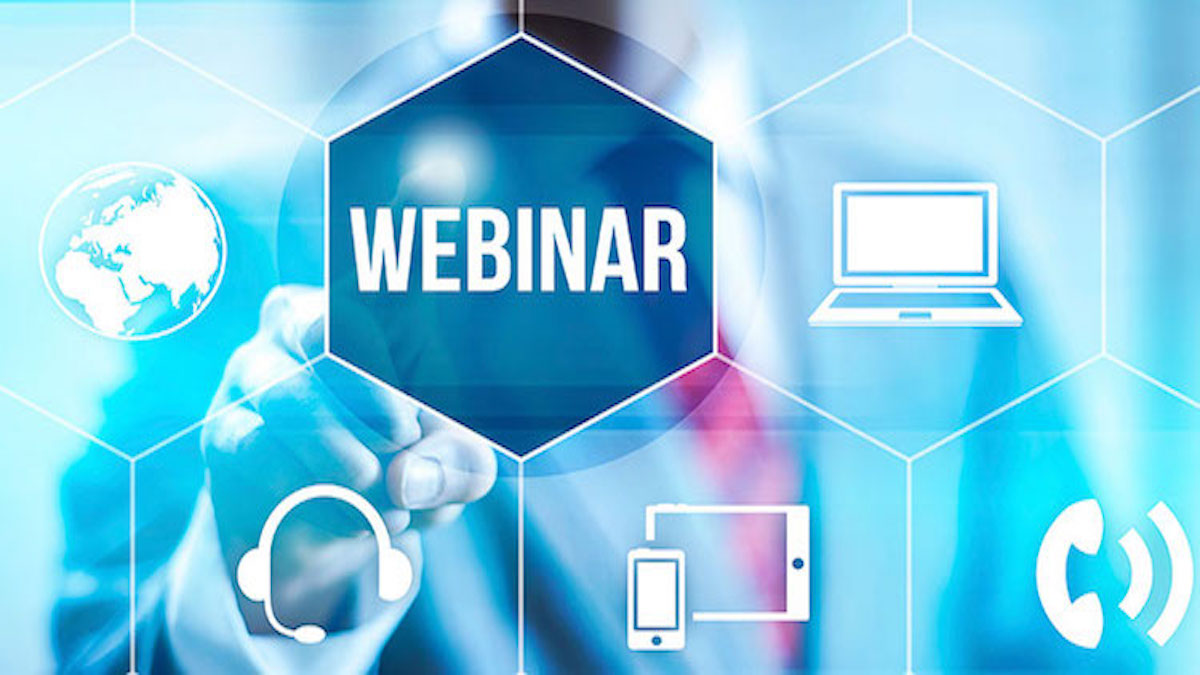
This is the 2nd session:
“MLOps”, a term coined less than 5 years ago, is often touted as “DevOps for ML”. This is a fitting ‘working definition’ since for a while now the emphasis is on enabling cluster-based (cloud or on-prem), containerized, end-to-end processes. The ‘new wave’ of MLOps redefines the target as providing the chain-of-custody in these processes. In other words, the provenance of the transition from data to deployed models. The core-mission of MLOps tools is thus the abstraction of this target.
Peculiarly, missing from both current and new definitions is the laborious process of research and development required prior to creating an end-to-end pipeline. Evidently, one can ‘carve’ specific capabilities from existing MLOps tools for, i.e. research, but because the orientation is towards ‘production’, most of the abstractions will tend to be ill-fitting. Which ones are still valid, and can be used to construct productivity-enhancing research MLOps?
Putting the researcher and her ability to experiment at the center, we recognize that the computational needs are unchanged from other disciplines: The need to orchestrate a large number of interdependent trials, in a reproducible and manageable way. The MLOps tools that enable this - are the most fundamental ones. From such, the road from research to production can be navigated using robust pipelines.
In this “best-practices” webinar, we will overview the “must-haves” and show examples of research-MLOPs that stand out: From easy remote execution, through magically reproducible setups, and even custom, reusable, research pipelines.
All sessions of the series: